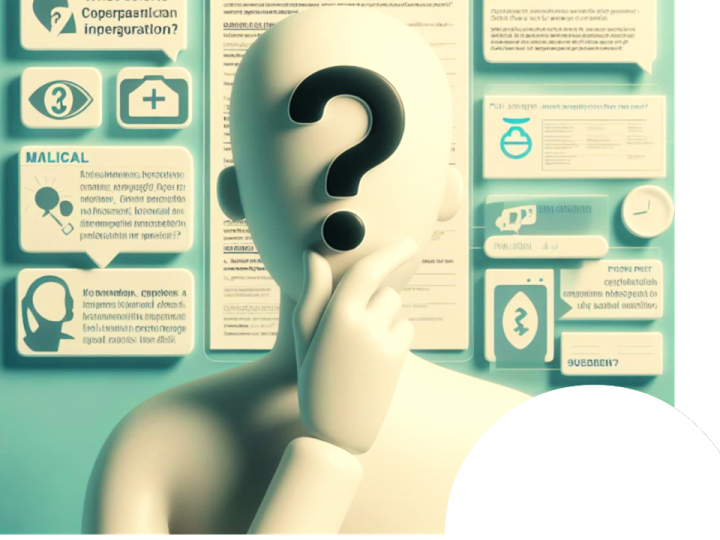
Semantics of Clinical Language
Hospitals amass crucial textual data for healthcare, often in disorganized forms within Electronic Health Record (EHR) systems. The semantics of language used in such texts differs a lot from ordinary English. Understanding the domain-specific semantics has consequential applications in streamlining health data, reducing redundancy, identifying errors, and retrieving and preserving valuable information.
In the modern world of increasingly digitized healthcare infrastructure, clinical notes and other reports are maintained digitally. They are, however, almost always manually created. This has resulted in pervasive copy-paste actions across the board, leading to an immense amount of redundant information in such notes and reports. Using an ensemble of traditional ontology-based methods and state-of-the-art neural networks, we developed a a lightweight but highly accurate system to detect clinical texts for semantic duplication and similarity (Salek Faramarzi et al.; 2022). Beyond identifying redundant information, the detection of medical events described in unstructured clinical notes is, arguably, of fundamental importance in healthcare. This is an extremely challenging task, as these events are described in complex narratives. We address this challenge by employing the Contextualized Medication Event Dataset (CMED) as part of our participation in the 2022 National NLP Clinical Challenges (n2c2) shared task. Our work evaluates the performance of various pretrained language models, reveals that data augmentation coupled with domain-specific training provides notable improvements (Salek Faramarzi et al.; 2023).
Team
- Ritwik Banerjee, Research Assistant Professor of Computer Science, Stony Brook University
- Noushin Salek Faramarzi, Research Assistant
- Akanksha Dara, M.S. ↦ Software Engineer, Apple Inc.
- Meet Patel, M.S. ↦ Software Engineer, Yahoo
- Sai Harika Bandarupally, M.S. ↦ Software Engineering Intern, Goldman Sachs
- Context-aware Medication Event Extraction from Unstructured Text. Proceedings Of The 5Th Clinical Natural Language Processing Workshop. https://doi.org/10.18653/v1/2023.clinicalnlp-1.11 (Original work published 2023). (2023).
- Combining Attention-based Models with the MeSH Ontology for Semantic Textual Similarity in Clinical Notes. 2022 Ieee 10Th International Conference On Healthcare Informatics (Ichi). https://doi.org/10.1109/ICHI54592.2022.00023 (Original work published 2022). (2022).